Alphabet’s subsidiary DeepMind has all but solved the problem of predicting a protein’s shape from its amino acid sequence. DeepMind’s program AlphaFold reached close to 90% average accuracy, which is comparable to experimental structural analysis.
Proteins: life’s multitool
Proteins are the primary tool with which organisms do stuff. They are indispensable for most biological processes. Proteins act as catalysts in chemical reactions, transport and store other molecules, give cells their structural integrity, transmit neural impulses, and provide us with immune protection. It was recently discovered that a single cell contains around 42 million protein molecules [1].
There are close to 20,000 protein coding genes in the human genome, but it is unclear exactly how many protein species they produce, since a single gene can make more than one protein due to alternative splicing. However, all this abundance stems from a mere 20 building blocks that are our amino acids. Transcribing DNA produces a string of messenger RNA (mRNA), which is then translated inside a protein factory (ribosome) into a sequence of amino acids: the nascent protein. As this long chain of hundreds or thousands of amino acids is leaving the ribosome, it begins to fold into an intricate 3D shape in accordance with chemical bonds that develop between its building blocks. There are recurrent structural elements, such as helices and pleated sheets, and the final shape is full of hairpin turns reminiscent of a mountain road. For example, this image might look like modern art, but it is the 3D structure of DNA polymerase II:
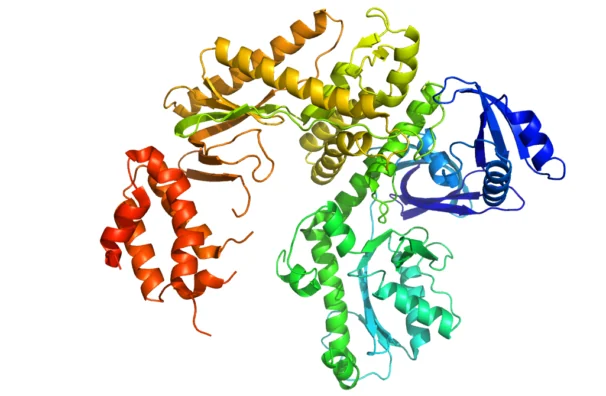
A protein’s function depends on its shape, and antibodies bind to antigens because they have matching shapes. Protein shapes vary from simple to extremely complicated, such as ATP synthase protein complex, a major element of energy production in the cell. It is a turbine-like machine that produces mechanical motion when hit by passing protons.
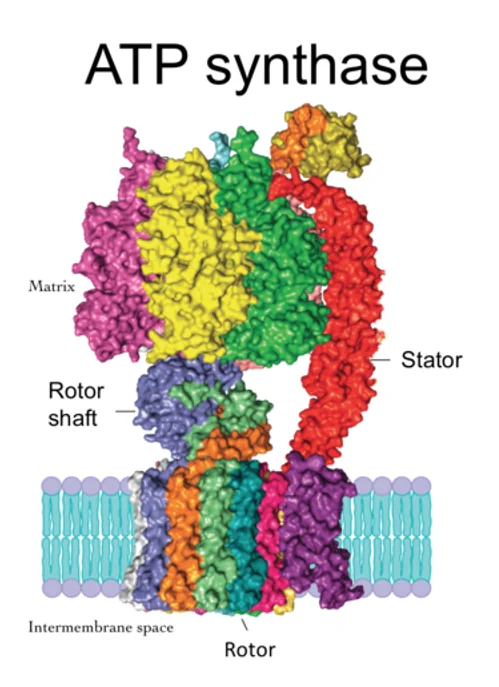
The protein-folding problem
To understand how the body works, we need to decode the shapes of its proteins. Scientists have been doing this for more than half a century now, using techniques such as X-ray crystallography and, more recently, cryo-electronic microscopy. However, this is a tedious process that can take months in a lab, with some especially stubborn proteins guarding the secrets of their shapes for years.
The other way would be to predict a protein’s shape solely from its amino acid sequence, which has become known as the protein-folding problem [2]. Attempts have been made since the 60s to crack this problem using computers, with limited success. In 1994, the Critical Assessment of Structure Prediction (CASP) biennial challenge was instituted, but while new computational methods have been able to predict structures of some simpler proteins, the more complex ones remained unassailable until recent advances in artificial intelligence.
Although AI initially gave CASP a bump, the progress had been stalled for years until 2018, when the field, previously occupied by small academic groups, was disrupted by a new major player: DeepMind, a subsidiary of Alphabet, Google’s umbrella company. DeepMind entered the public consciousness after its creation, the AlphaGo program, had defeated some of the world’s strongest Go players. AlphaFold, another DeepMind product, established itself at CASP-13 in 2018 as a master protein shape predictor, demonstrating considerable improvement over earlier attempts. For this year’s CASP-14, DeepMind thoroughly reworked AlphaFold, which now correctly predicts an average of around 90% of a protein’s structure, as Nature has graphed:
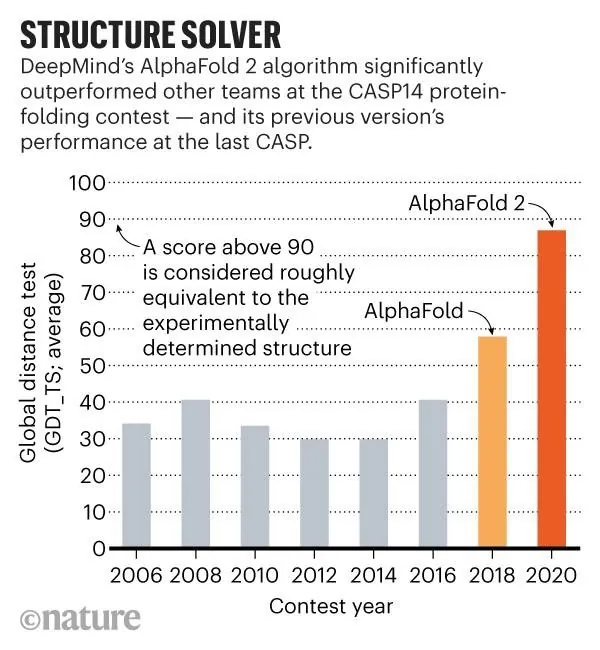
This result is comparable to experimental structural analysis, which makes AlphaFold 2 a true gamechanger. The team has not yet published an accompanying paper, but here is the link to the 2018 paper.
Two other tech giants, Microsoft and China’s Tencent, participated in CASP-14, though their solutions were less successful. Notably, in 2018, DeepMind made core data about AlphaFold widely available, allowing other teams to benefit from it. This may be the reason why this year, the average team score was higher than DeepMind’s record-breaking result of 2018.
What now?
The possible applications for computerized protein structure prediction are many. First, it is way faster than the conventional methods of structural analysis. At the beginning of this year, AlphaFold predicted the structure of several SARS-CoV-2 associated proteins long before the experimental results came in. This can make a big difference, especially with rapidly mutating viruses. AlphaFold can reveal the structures of certain proteins that defy experimental methods, such as membrane proteins that are notoriously hard to crystallize. AlphaFold can also be used to reliably create new proteins with previously unseen functions that can become foundations for drugs. It can provide new clues relating to the problem of protein misfolding, which plays a major role in neurodegenerative diseases [3]. Finally, DeepMind’s new wonder child can be used for designing protein-based nanomachines, not unlike ATP synthase. Previous attempts included techniques such as DNA origami, but protein folding technology may one day create structures that interface with our cells in ways never before thought possible.
Literature
[1] Ho, B., Baryshnikova, A., & Brown, G. W. (2018). Unification of protein abundance datasets yields a quantitative Saccharomyces cerevisiae proteome. Cell systems, 6(2), 192-205.
[2] Dill, K. A., & MacCallum, J. L. (2012). The protein-folding problem, 50 years on. science, 338(6110), 1042-1046.
[3] Sweeney, P., Park, H., Baumann, M., Dunlop, J., Frydman, J., Kopito, R., … & Hodgson, R. (2017). Protein misfolding in neurodegenerative diseases: implications and strategies. Translational neurodegeneration, 6(1), 6.