A group of scientists has created a neural network that identifies senescent cells by their morphology and can be used for senolytic drug screening [1].
Image recognition is a showcase triumph of artificial intelligence. While this problem was considered extremely hard to crack in previous decades, algorithms have now exceeded human abilities in multiple cases. Automated image recognition has already been employed in medicine. For instance, algorithms are better at recognizing some malignant tumors than human radiologists [2].
Computers owe their success in this and many other fields to machine learning: the ability of machines to learn from data and get constantly better at virtually anything. Most machine learning algorithms rely on neural networks, which are inspired by the way the human brain works. In this new paper, a group of Japanese scientists describes an intriguing new application for automated image recognition: determining whether a cell has gone senescent.
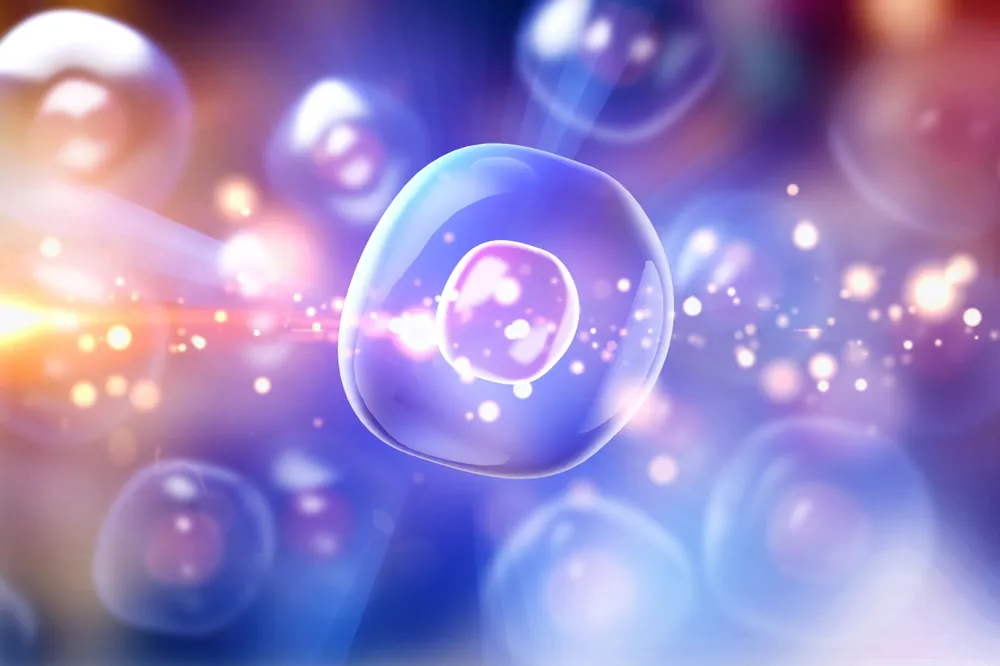
Read More
The shape of senescence
When talking about cellular senescence, we usually refer to the cell’s operation and chemical output, but senescence also changes the cell’s morphology. For instance, healthy epithelial cells are elongated but upon entering senescence they acquire a rounder shape.
In this paper, researchers have shown that a fairly basic convolutional neural network (CNN) can correctly predict if a cell has gone senescent solely from its shape. CNNs are widely used for image recognition because their operation is similar to our own vision. They start with recognizing the simplest patterns (a straight line, a dot, etc.) and work their way up several layers of virtual neurons, recognizing increasingly more complex parts of the whole image. Simply speaking, we recognize a face by identifying its elements first, and this is also how CNNs work.
Cellular senescence can be induced by various internal and external factors. To make the model more robust, the researchers used two types of senescence inducers: oxidation stress (by applying oxygen peroxide) and an anti-cancer drug (camptothecin). CNNs were trained to distinguish between individual images of senescent cells and of healthy control cells obtained by phase-contrast microscopy. The researchers trained three CNNs, one on each type of a senescence inducer and an additional one on both types. Each CNN was then tested on data that included both of those types along with cells whose senescence was induced by replicative stress. In each combination, the accuracy of prediction hovered around a respectable 90%. The researchers called their model Deep Learning-Based Senescence Scoring System by Morphology (Deep-SeSMo).
The scientists also established that Deep-SeSMo can work with images obtained at another institution, Kyoto University. While all CNNs did the job well, the best results, unsurprisingly, were demonstrated by the CNN that had been initially trained on the datasets from both institutes.
Then, the group examined whether Deep-SeSMo could identify senescence in a different cell type. Initially, the authors used human umbilical vein endothelial cells (HUVECS), but Deep-SeSMo was just as successful when trained and tested on human diploid fibroblasts (HDFs). Interestingly, CNNs trained on HUVEC datasets were also able to recognize senescent HDFs. This suggests that senescent cells of various types share certain morphological characteristics that can be identified by a morphology-based CNN.
Looking for new drugs
The researchers suggest that this method can be used in senolytic drug screening. As a proof of concept, they screened several substances known as either senescence-suppressing, senolytic, or senescence-neutral. Nicotinamide mononucleotide (NMN) and metformin are known to suppress senescence, and Deep-SeSMo successfully assessed their effect on the ratio of senescent cells in the tissue. Deep-SeSMo also correctly identified the senolytic effect of the ABT263 drug and correctly flagged a few substances as lacking any senescence-related activity.
Finally, the researchers used a kinase inhibitor library to look for new compounds that suppress cellular senescence. Of the several compounds tested, terreic acid, a metabolite of the fungus Aspergillus terreus, known for its anti-bacterial properties, showed the most promise. The authors suggest that terreic acid can be a viable candidate senolytic.
Conclusion
This research shows that machine learning techniques can bring inspiring results when applied to problems in the medical field. The amalgamation of conventional medicine with big data and machine learning technologies is already happening, and it will keep yielding breakthroughs as biologists become more open to novel computational methods and more tech companies enter the field. For a deeper dive into AI and medicine, read our recent review of Tina Wood’s book, “Live Longer With AI”.
Literature
[1] Kusumoto, D., Seki, T., Sawada, H., Katsuki, T., Kimura, M., Ito, S., … & Yuasa, S. (2020). Anti-senescent Drug Screening by Deep Learning-based Morphology Senescence Scoring. Circulation, 142(Suppl_3), A13576-A13576.
[2] Boulos, M. N. K., Peng, G., & VoPham, T. (2019). An overview of GeoAI applications in health and healthcare.